Okay, so today I’m gonna walk you through my little adventure with “lena theis”. I ain’t no expert, just a regular guy messing around with stuff, so bear with me!
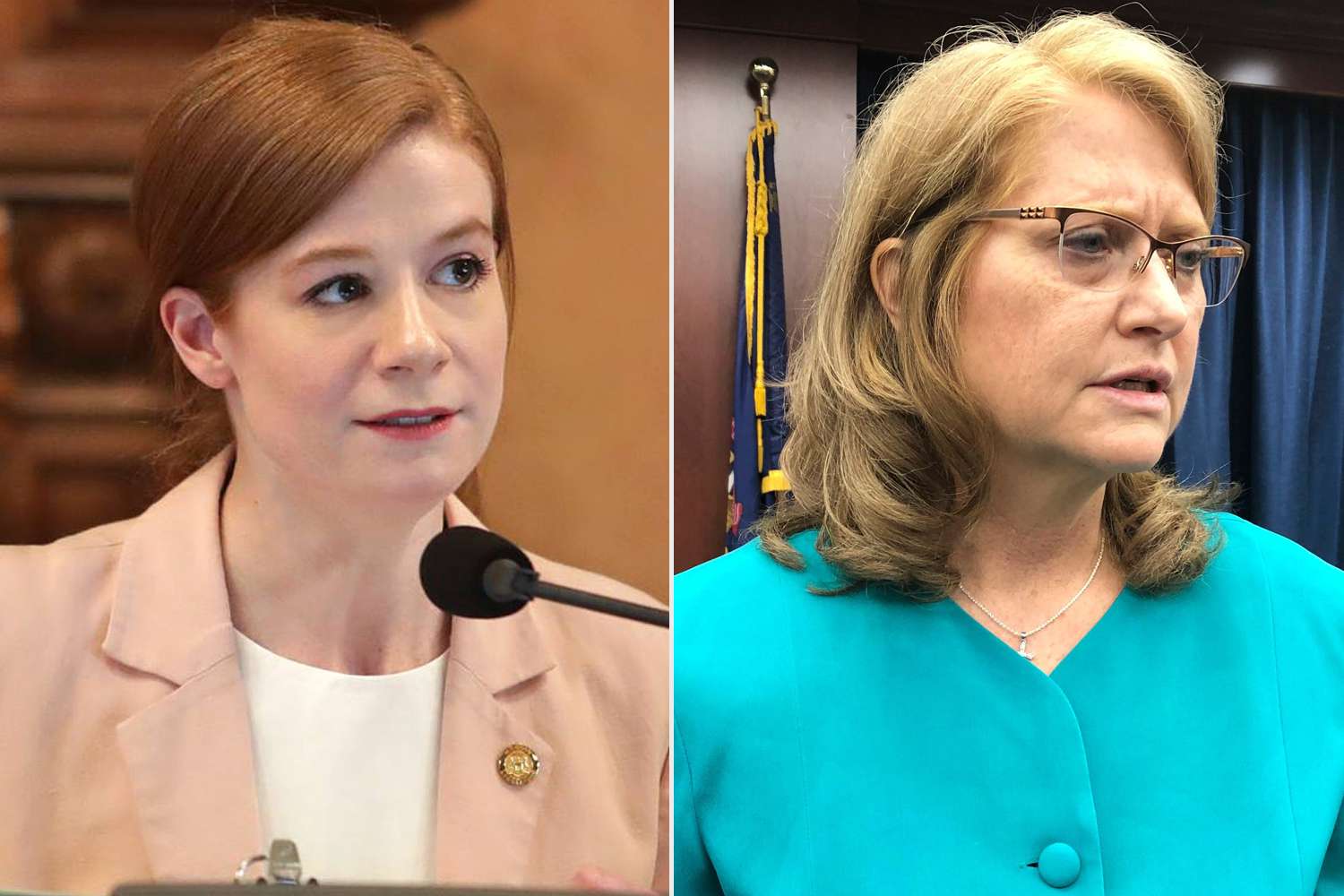
First things first, what’s “lena theis”? I stumbled upon it while looking for some cool datasets to play with. Heard it was a good one for learning about single-cell RNA sequencing data. Looked interesting, so I figured, “Why not?”.
So, I started by downloading the dataset. It’s pretty big, like several gigabytes, so that took a while. While that was downloading, I installed scanpy. Scanpy’s like this Python library, super useful for analyzing single-cell data. Just a quick pip install scanpy
in my terminal, and I was good to go.
Once the download was complete, I loaded the data into scanpy. Something like adata = *_h5ad("path/to/your/lena_theis.h5ad")
. It felt like opening a treasure chest, I was pretty excited to see what’s inside.
Then came the fun part: exploring the data. I started with some basic stuff like and to check out the metadata. It’s like looking at the labels on all the boxes in that chest. Needed to know what was what, right?
Next, I did some quality control. Single-cell data can be noisy, with dead or messed up cells screwing things up. So, I had to filter out cells that didn’t meet certain criteria. This is where Scanpy shines, you can calculate metrics like the number of genes expressed per cell, or the percentage of mitochondrial genes, and then remove cells with weird values.
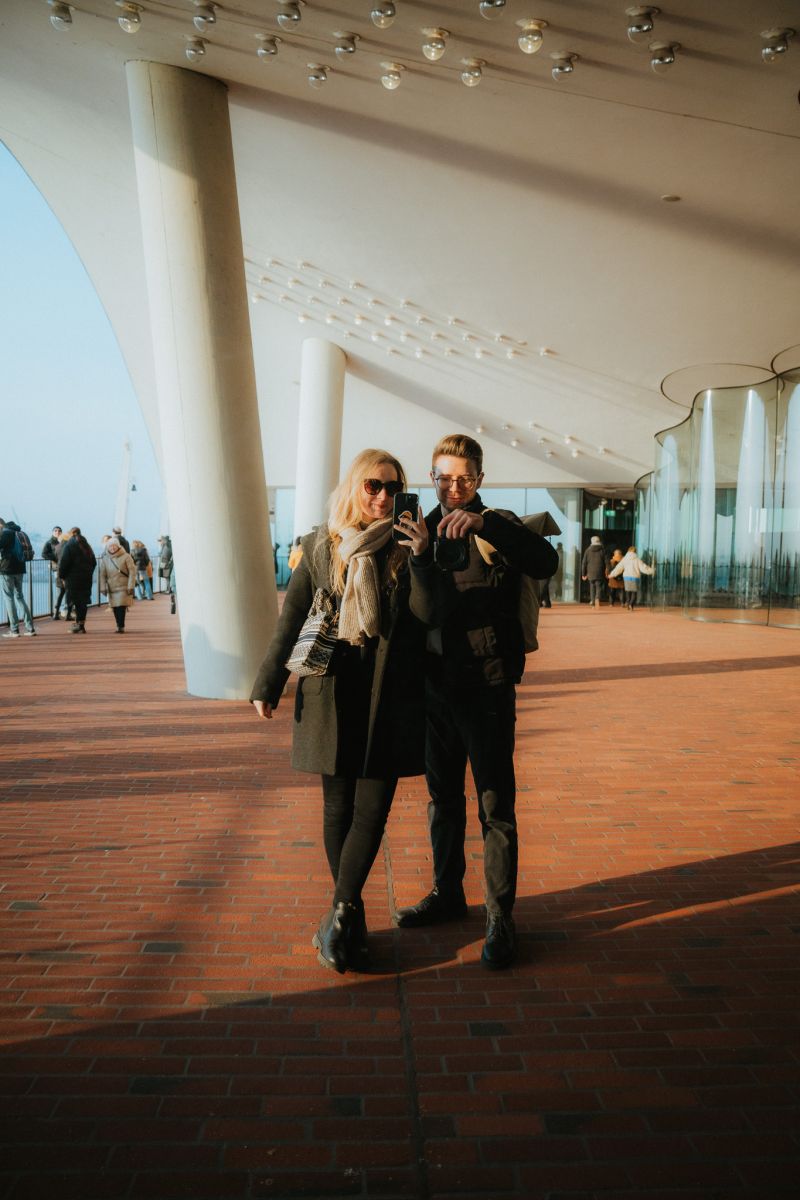
After cleaning up, it was time to normalize and scale the data. This makes sure that all the cells are on a level playing field, so one cell’s high expression of a few genes doesn’t overshadow everything else. Scanpy has functions like *_total
and for that.
Then, I reduced the dimensionality of the data. All those genes, all those cells – it’s a lot to process! Dimensionality reduction techniques, like PCA, help boil down the data to its most important features, making it easier to visualize and analyze. to the rescue!
Now, the really cool part: visualizing the data. I used UMAP (Uniform Manifold Approximation and Projection) to project the cells into a 2D space. Cells that are similar to each other end up close together. Ran and then . After that I got a fancy plot using , each dot representing a cell. It’s awesome to see all the cells arranging themselves into clusters!
Finally, I tried to identify cell types. By looking at the expression of known marker genes, genes that are specific to certain cell types, I tried to figure out what each cluster of cells represented. This part’s tricky, takes a lot of knowledge and sometimes you’ve gotta do some digging to find the right markers. But it’s super rewarding when you start to make sense of the data!
What did I learn? Well, for starters, single-cell data analysis is no walk in the park. It’s a lot of steps, a lot of tweaking, and a whole lot of reading documentation. But it’s also incredibly powerful. You can uncover hidden patterns in the data, identify rare cell types, and get a better understanding of how cells work. It was a fun little project. I might try a different dataset next time!
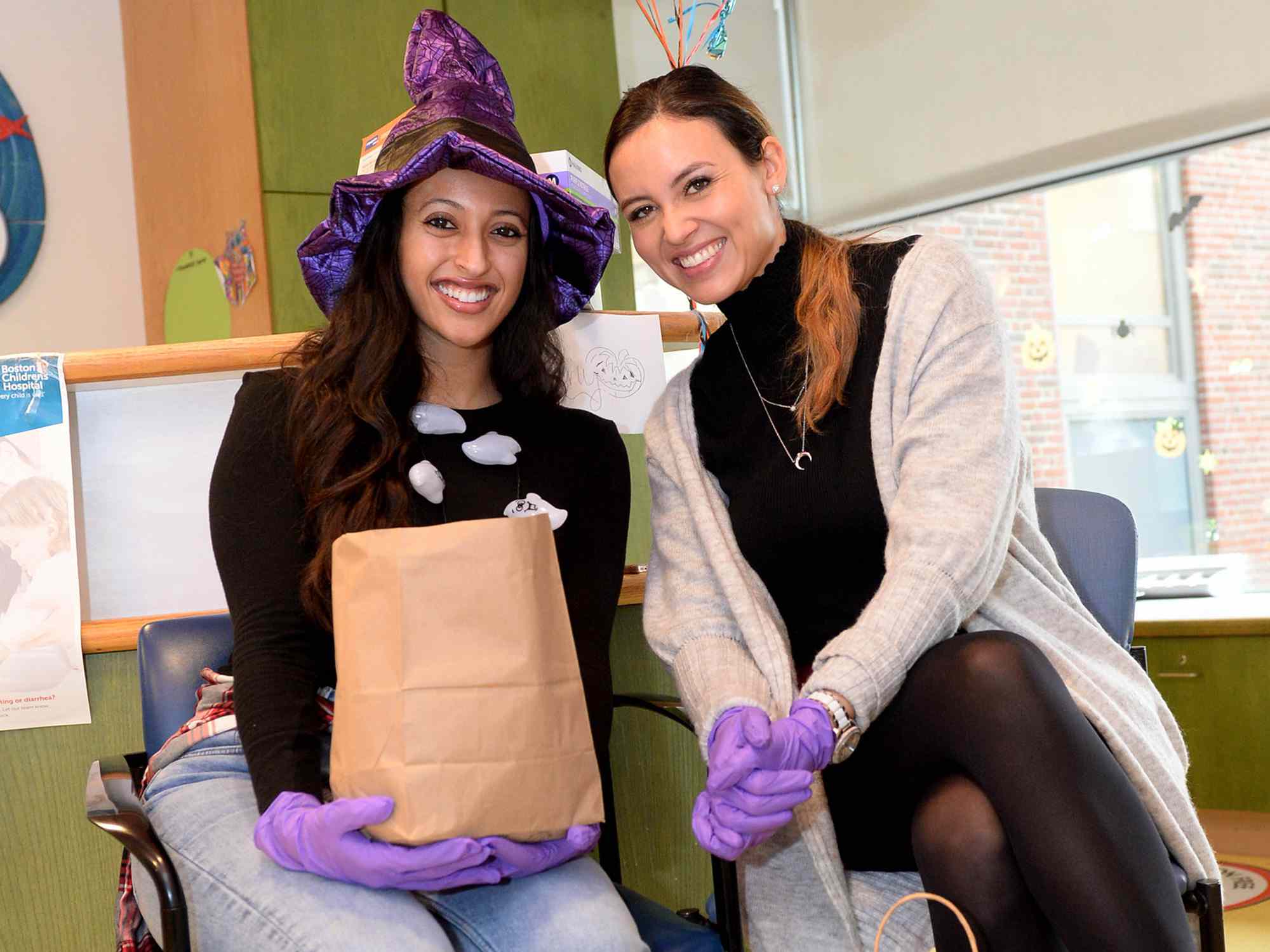