Alright, so today I’m gonna walk you through my little adventure with “dylan edwards.” No fancy stuff, just how I messed around and what I learned. Buckle up!
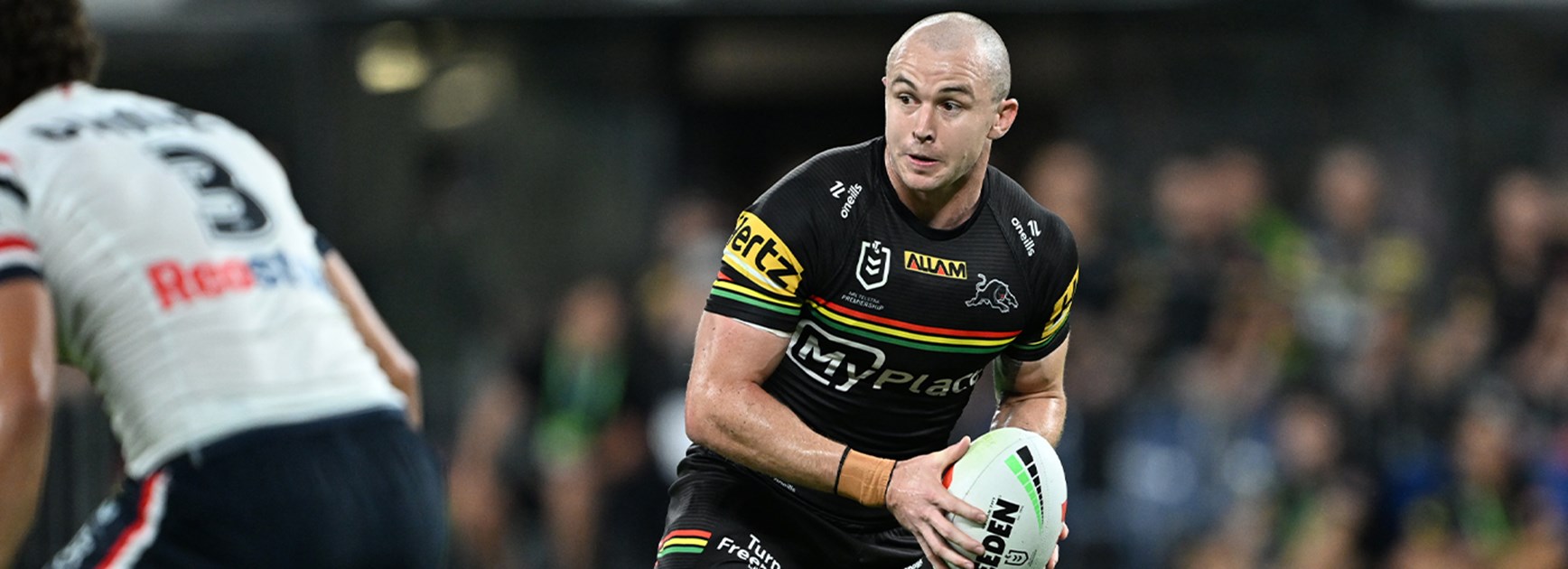
First off, I heard the name “dylan edwards” floating around. Sounded interesting, so I figured, why not poke around a bit? My starting point was pretty basic – just a quick Google search. Nothing groundbreaking, but hey, gotta start somewhere, right?
So, I start digging, right? I mean, really digging. I’m looking at different angles of approach and stuff. I decided to try something a little different, I thought maybe a specific angle was what was needed. So what do I do? I hop onto some forums, see what the chatter is like, try to find some leads.
I spent a good chunk of time reading through forum threads, blog posts, and even a few academic papers. It was a bit of a rabbit hole, I’m not gonna lie. Lots of stuff that didn’t quite click, but every now and then, a little nugget of info would pop up. I took notes like crazy, trying to piece everything together. Think of it like a really messy jigsaw puzzle.
The breakthrough came when I stumbled upon a mention of a particular dataset. Turns out, “dylan edwards” was connected to this big pile of information. Score! I downloaded the dataset and started cleaning it up. Man, that was a chore. Missing values, weird formats – you name it, it was there. But I pushed through, because I had to.
With the dataset cleaned up, I started running some basic analyses. Nothing too fancy – just some descriptive stats and visualizations. But even that gave me some interesting insights. I started seeing patterns and relationships that I hadn’t noticed before. It was like the picture was slowly coming into focus.
Next up, I decided to try my hand at modeling. I built a simple model and ran it on the dataset. The results were… okay. Not amazing, but not terrible either. More importantly, it gave me a baseline to work from. I tweaked the parameters, tried different algorithms, and slowly but surely, the model started improving.
Here’s where things got interesting. I started digging into the specific cases where the model was making mistakes. Turns out, there were some subtle nuances in the data that I had missed. Things like contextual factors and hidden variables. I went back to the dataset and started cleaning it up. Man, that was a chore. Missing values, weird formats – you name it, it was there. But I pushed through, because I had to.
The biggest challenge was dealing with the noise. There was so much irrelevant information in the dataset that it was hard to separate the signal from the noise. I spent a lot of time filtering and cleaning the data, trying to isolate the key variables. It was tedious, but it paid off in the end.
Along the way, I hit a few roadblocks. There were times when I just couldn’t figure something out. I’d bang my head against the wall for hours, trying to solve a problem. But eventually, I’d either find a solution or realize that I was barking up the wrong tree. It’s all part of the process, I guess.
- First I started searching.
- Second was find a dataset.
- Third I cleaned the dataset.
- And the last thing was model the dataset.
After a lot of trial and error, I finally got to a point where I was happy with the results. The model was performing well, and I had a pretty good understanding of what was going on. I wrote up a report summarizing my findings and shared it with a few colleagues. They gave me some feedback, which I incorporated into the final version.
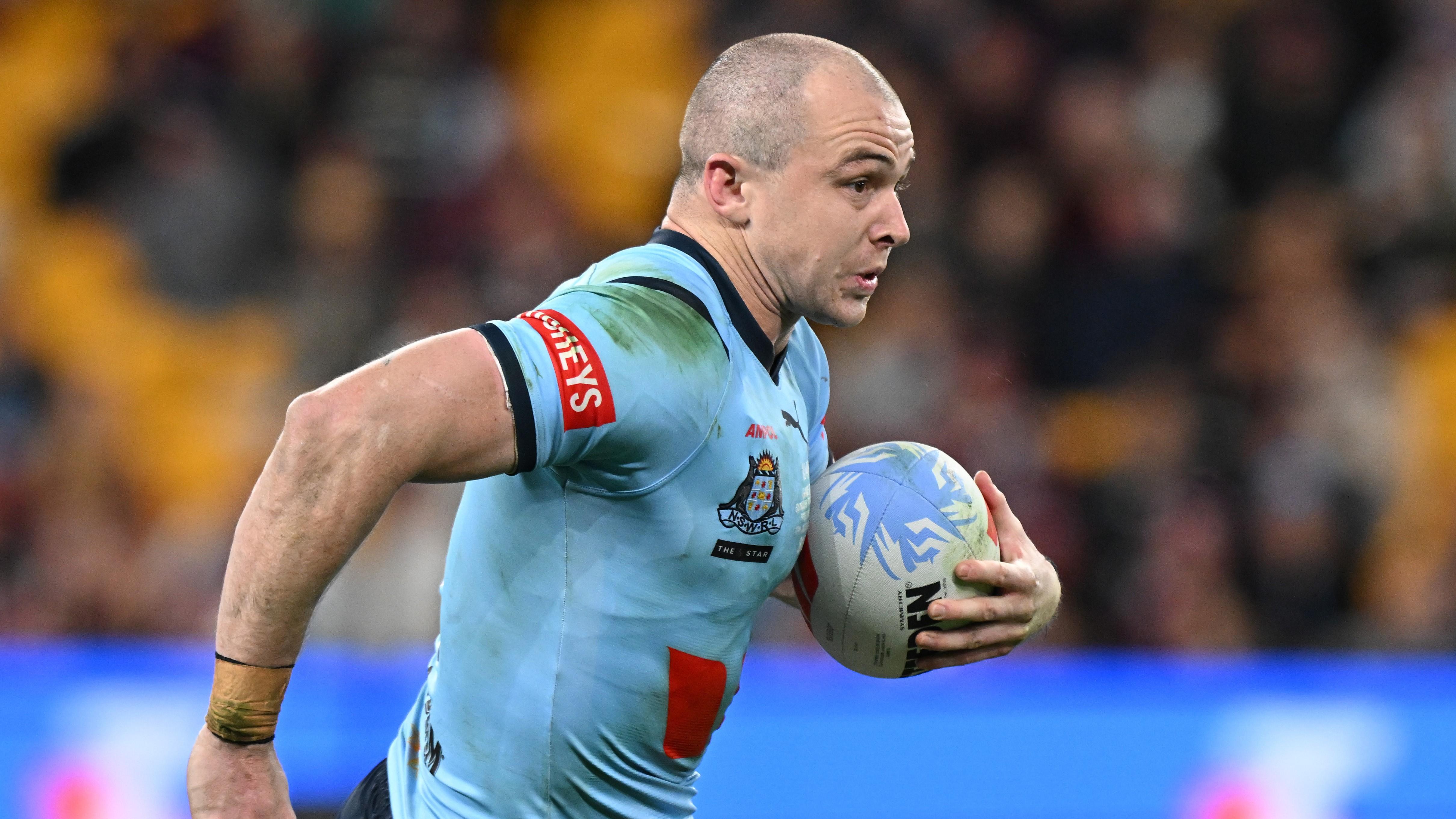
So, that’s my “dylan edwards” story. It wasn’t easy, but it was definitely worth it. I learned a lot about data analysis, modeling, and the importance of perseverance. And who knows, maybe it’ll inspire you to go on your own data adventure. Go for it!
What I learned
- Data is messy
- Persistence is key