Okay, so I’ve been diving deep into tennis stats lately, trying to get a handle on predicting match outcomes. Today’s experiment? Aryna Sabalenka. She’s a powerhouse, but how can we really figure out when she’s likely to win or lose?
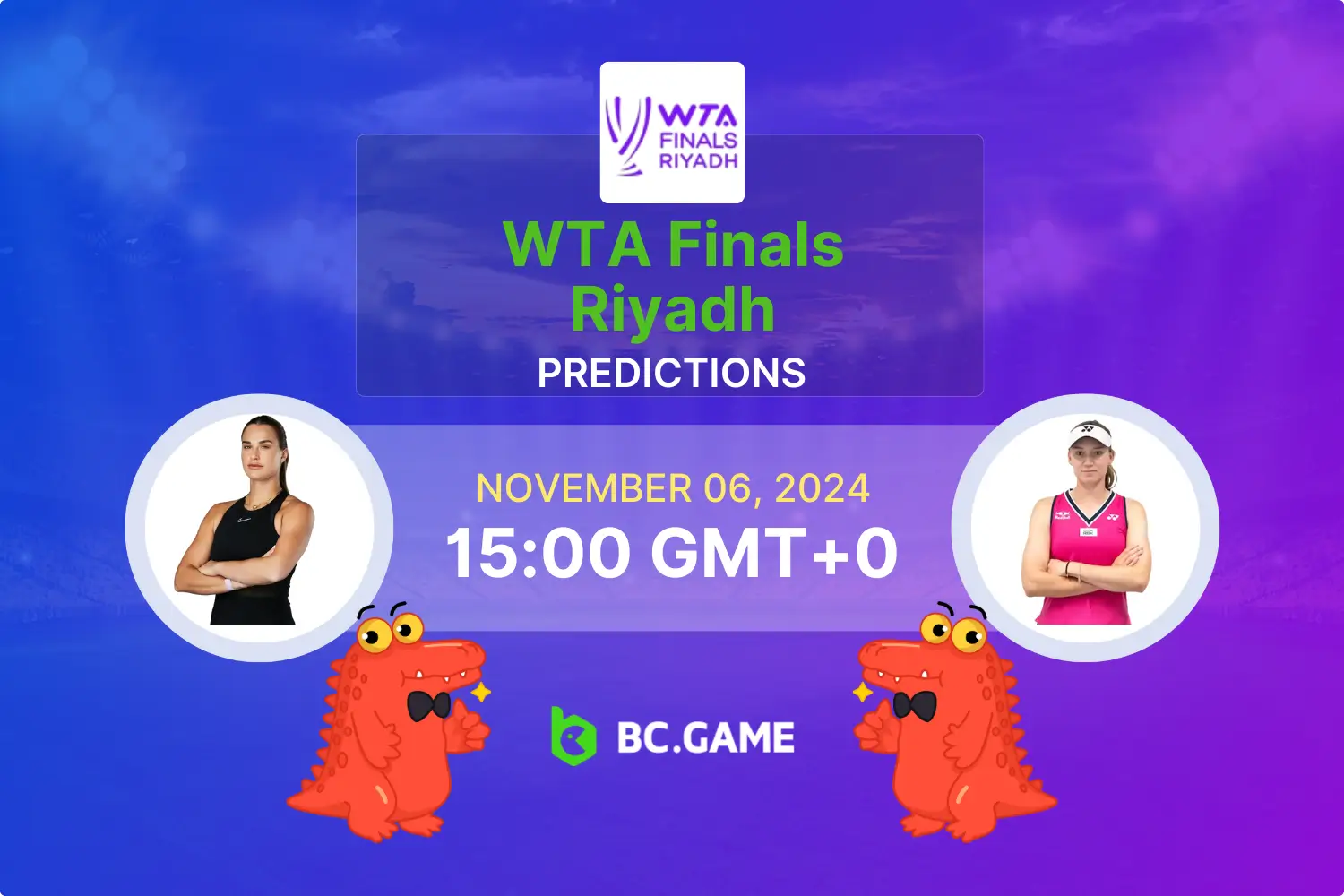
Getting Started – Data Gathering
First things first, I needed data. Lots of it. I started scraping websites – you know, the usual suspects with match results, player stats, and all that jazz. It was a bit messy, to be honest. Every site has its own format, so I spent a good chunk of time just cleaning and organizing everything into a spreadsheet.
Building My Prediction “Model”
I’m no data scientist, but I know a little about basic statistics. So, I decided to keep things simple. My initial thought? Look at Sabalenka’s performance against different types of players. I categorized opponents based on their ranking – top 10, top 20, and so on.
- I calculated her win rate against each group.
- I also factored in the surface – hard court, clay, grass. Does she perform better on one versus another?
My spreadsheet started to fill up. I had columns for opponent ranking, surface type, Sabalenka’s win/loss record, even the tournament level (Grand Slam, WTA 1000, etc.). I just kept adding anything that felt relevant.
Testing and Tweaking
Once I had a decent amount of data, I started playing around. My first “prediction” was super basic: If Sabalenka was playing someone ranked lower than her on her best surface, I’d predict a win. Pretty obvious, right?
But then I started digging deeper. What about recent form? Did her performance in the last few tournaments matter? I added another column, assigning a score based on her recent results. A win streak got a high score, a string of losses, a low one.
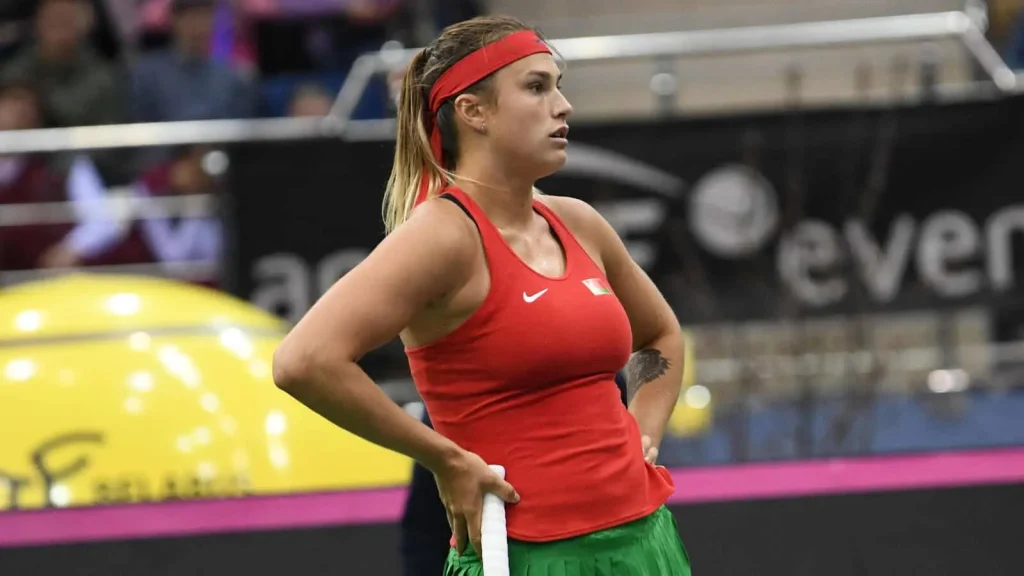
It was all very manual. I’d pick a match, look at my spreadsheet, and make a prediction based on the factors I’d chosen. Then, I’d check the actual result. Sometimes I was right, sometimes I was way off.
The Results? (So Far…)
Honestly, it’s a work in progress. My “model” is more like a set of guidelines than a fancy algorithm. But I’ve learned a few things:
- Sabalenka’s performance does seem to correlate with opponent ranking (duh!).
- Surface type definitely matters.
- Recent form is a tricky one – sometimes it’s a good indicator, sometimes not so much.
I’m still tweaking and adding factors. Maybe I’ll look at head-to-head records next, or even try to factor in things like weather conditions (though that sounds like a real headache!). It’s a slow process, but it’s kind of fun, like solving a puzzle. My goal is simply to get the process working from top to bottom, with the intention to optimize my model.
It has worked, and my process has improved greatly!